Ph.D. , Information Systems, 2022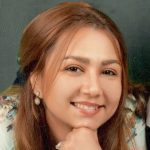
![]()
Dr. Ronak Razavisousan was advised by Dr. Karuna Joshi from 2018-2022. Her research interests lie in the broad area of Data Science.
She developed a novel methodology, called Textual Fuzzy Interpretive Structural Modeling (TFISM), that automatically analyses large textual datasets to identify the internal and external relationships between factors in the document. She applied her methodology to analyze documents in various domains like Supply Chain Management, Data Privacy regulations and Student Mobility.
She is currently an Assistant Professor at Coppin State University. She has also worked as a Senior Data Scientist at Stonehenge.
Publications
- R. Razavisousan and K. P. Joshi, “Analyzing GDPR compliance in Cloud Services’ privacy policies using Textual Fuzzy Interpretive Structural Modeling (TFISM)“, In Proceedings of IEEE International Services Computing Conference (IEEE SCC) 2021.
- R. Razavisousan and K. P. Joshi, “Building Textual Fuzzy Interpretive Structural Modeling to Analyze Factors of Student Mobility“, TechReport, UMBC, April 2021
- Ronak Razavisousan and Karuna P. Joshi, “Two Tier Analysis of Social Media Collaboration for Student Migration“, In Proceedings of IEEE International Conference on Collaboration and Internet Computing (IEEE CIC), 2019
Ronak successfully defended her Ph.D. Thesis, Building Textual Fuzzy Interpretive Structural Modeling to Analyze Complex Problems, in September 2022.
Ronak successfully defended her Ph.D. Proposal in March 2020.
Proposal: Developing Student Mobility Model using Fuzzy Interpretive Structural Modeling (Fuzzy- ISM) for Social Media data
Committee: Dr. Karuna P Joshi (Chair), Dr. Zhiyuan Chen, Dr. Vandana Janeja, Dr. George Karabatis, Dr. Yelena Yesha
Abstract: Economists, sociologists, and general social scientists have attempted to clarify the human migration concept. Their success is influenced by changes in general society because of the internal relationships between pulling, pushing, and emerging new factors. In recent years, technology enhancement and globalization caused a drastic increase in human migration all over the world. At the same time, host countries began focusing on educated immigrants and the benefits they afforded their nations. Modern methods for studying human behavior, such as social media analysis and computational analysis, are not effectively employed in the field of human migration and specifically student mobility. Our research explores student mobility motivations, characteristics, competencies, and approaches through social media data with the help of Natural Language Processing (NLP) techniques. This work tries to identify the factors of student mobility and highlight the role and weight of each one, to model the transition of a student in different situations. The relationship between these factors fills the dearth of knowledge concerning human migration focusing on student mobility.
This research primarily utilizes quantitative methods, but where clarification or detail is needed a qualitative method is implemented. The proposed solution is conducted in two parts. In the first part, the collected data from social media (Twitter) is examined with an established economic migration model. NLP techniques boost the content analysis and find the similarity between datasets. In the second part, I model the factors extracted from the data with the help of the prevailing modeling methodology. A fuzzy extension of Interpretive Structural Modeling (Fuzzy-ISM) is customized for textual data and the process flow designed to run the model automatically.
Ronak was invited to present at the Data Science Consortium organized by Michigan Institute for Data Science (MIDAS) on November 13-15 2019.