MS, Computer Engineering, May 2019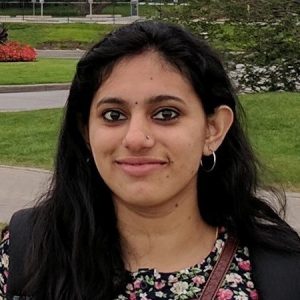
Arya Renjan worked on the IP Reputation scoring model project.
Along with KNACC lab, she was also a part of Ebiquity Research group.
Arya is currently working for Cisco. Prior to UMBC, she worked with ANSYS Inc. for three years, a tier-1 EDA company in the field of Semiconductor Simulation Software at Bangalore, India.
She successfully defended her Master’s thesis in April 2019.
Thesis: A Policy-based Framework for Privacy-respecting Deep Packet Inspection in TLS Implementations
Committee: Dr. Karuna P Joshi (Chair), Dr. Anupam Joshi, Dr. Tim Finin, Dr. Mohamed Younis
Abstract:
Deep Packet Inspection (DPI) is instrumental in investigating the presence of malicious activity in network traffic, and most existing DPI tools work on unencrypted payloads. As the internet is moving towards fully encrypted data-transfer, there is a critical requirement for privacy-aware techniques to efficiently decrypt network payloads. Until recently, passive proxying using certain aspects of TLS 1.2 were used to perform decryption and further DPI analysis. With the introduction of TLS 1.3 standard that only supports protocols with Perfect Forward Secrecy (PFS), many such techniques will become ineffective. We have developed an ABAC (Attribute Based Access Control) framework that efficiently supports existing DPI tools while respecting user’s privacy requirements and organizational policies. It gives the user the ability to accept or decline access decision based on his privileges. Our solution evaluates various observed and derived attributes of network connections against user access privileges using policies described with semantic technologies. Network meta-characteristics like IP intelligence is one of the many attributes that may be used in defining access control policies. We also present Dynamic Attribute based Reputation (DAbR), a Euclidean distance based technique, to generate reputation scores for IP addresses by assimilating meta-data from known bad IP addresses. The reputation scores when used in conjunction with the policy enforcement module, can provide high performance and non-privacy-invasive malicious traffic filtering. We describe our framework and demonstrate the efficacy of our technique with the help of use-case scenarios to identify network connections that are candidates for Deep Packet Inspection. Since our technique makes selective identification of connections based on policies, both processing and memory load at the gateway will be reduced significantly.
Publications
- Arya Renjan, Karuna Pande Joshi, Sandeep Nair Narayanan, and Anupam Joshi, “DAbR: Dynamic Attribute-based Reputation scoring for Malicious IP Address Detection“, In Proceedings of IEEE Intelligence and Security Informatics (IEEE ISI) 2018