Ph.D. Candidate, Information Systems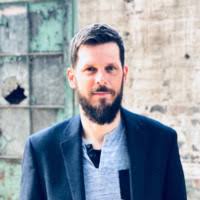
![]()
James Clavin is a Ph.D. Candidate at the University of Maryland, Baltimore County (UMBC) advised by Dr. Karuna Joshi. His general research interest is in distributed systems and consensus protocols and how they can improve healthcare data security, privacy, compliance, and interoperability through blockchain, Byzantine Fault Tolerance, and Health Informatics.
He is currently the Chief Technology and Compliance Officer of Hilltop Institute at UMBC.
Publications are available at Google scholar:
https://scholar.google.com/citations?user=oVprICwAAAAJ&hl=en&oi=ao
James successfully defended his Ph.D. Proposal on 1 December 2022.
Proposal: Integrating Knowledge Graphs With Byzantine Fault Tolerance for Insuring Compliance: Medical Data Polygraph
Committee: Dr. Karuna P Joshi (Chair), Dr. Sisi Duan (Tsinghua University), Dr. Patricia Ordoñez, Dr. Ian Stockwell, Dr. Jianwu Wang
Abstract: Healthcare organizations often have to exchange sensitive health records across peer-to-peer networks, and it is challenging to proactively find and fix compliance issues and incorrect data transfers. The Healthcare industry anticipates a growing need to identify faulty medical data when linking individuals’ electronic health records (EHR) across systems and to audit data having been shared outside the scope of data use agreements. We have developed a novel methodology that integrates semantic reasoners with Blockchain technology to track and correct health data exchange automatically. We propose a network layer that identifies errors in health information exchange between organizations that has at its core a two-layer Byzantine Fault Tolerant (BFT) protocol that uses smart contracts and machine learning to identify medical errors. Generally, we consider these Byzantine medical faults. Using instances of existing data use agreements and from the medical literature, we measure the effects of introducing errors into the system. We consider our identification and recommended fixes to such data to be insurance against error by enforcing compliance with regulation and enabling apparent cause analyses before adverse events occur.
Paper presented at the ACM DEBS conference